Data Lakes vs. Data Warehouses vs. LakeHouses
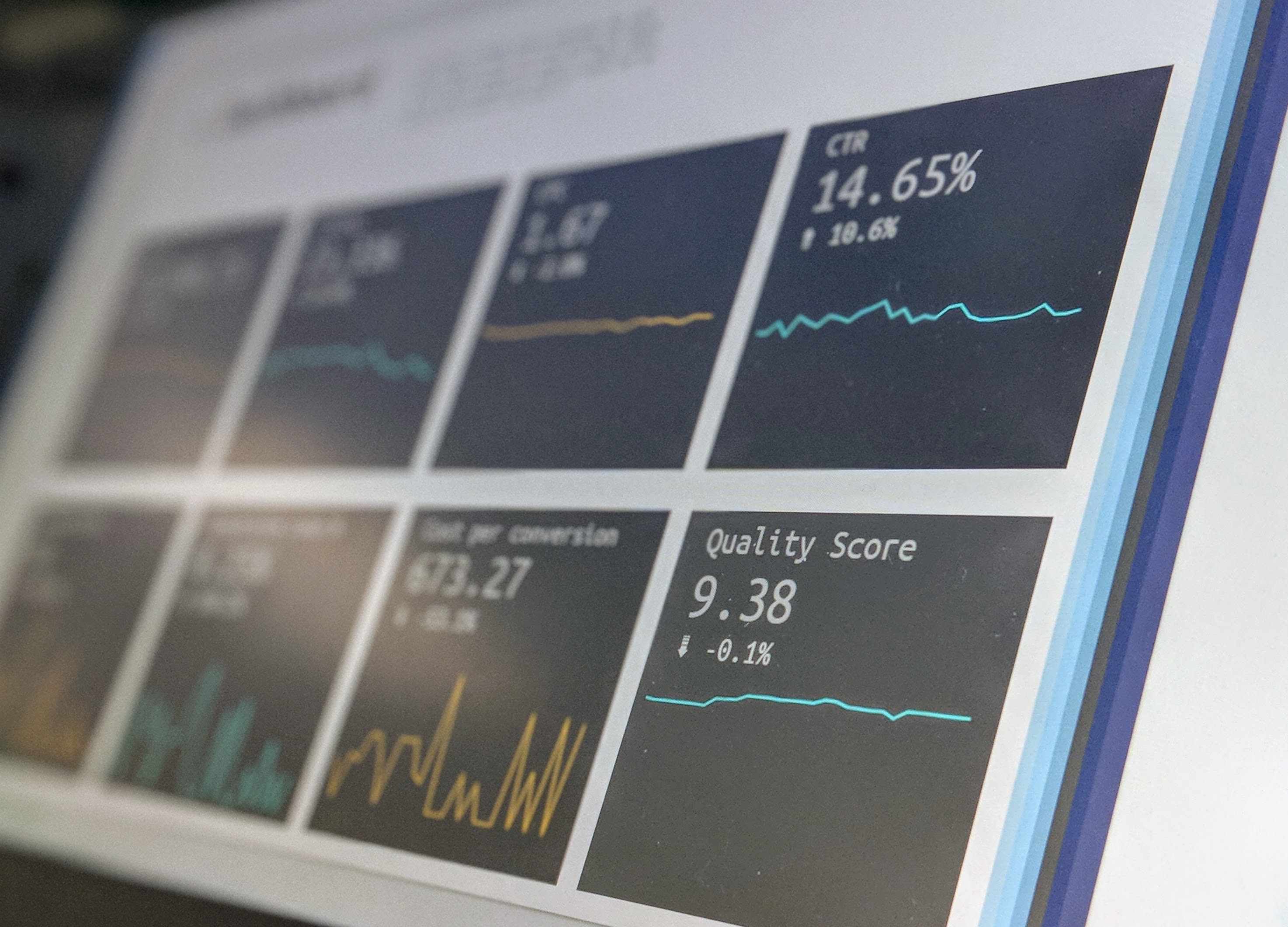
Before diving into the content that will be covered today, I should get the disappointment out ahead of time and share that this blog will not focus on beautiful waterfront views, or even giant warehouses with forklifts and product pallets abound. Rather, we at Kodda wanted to address the key differences between the way data is stored and how those differences affect how businesses can get the most of their data. So let’s get right into it with some definitions:
First we have a data lake. Think about this in terms of nature itself. Raw, undefined on its own, and no obvious structure. For a business, your data lake is the collection of your raw data which has yet to go through any ordering, and sometimes even cleansing. That doesn’t mean a data lake is without value though, but more on that later.
Next we have a data warehouse. This is when the data has been cleaned up and refined. In contrast to a data lake, we’ve now gone from atoms to molecules, and can view or extract sensible insights from out data. Most sales, marketing, and general business reporting falls into this category.
Lastly we have data lakehouses. Think of these as the combination of the former two. We’re now working with both unrefined data, as well as cleaned and modeled data.
Now…what really distinguishes these from one another, and as a business leader, when should you utilize each?
For data warehouses and lakehouses, you have ready-to-run insights that can provide immediate impact to your sales, marketing, and service functions of the business. Whether that’s reporting on customer LTV (lifetime value), sales KPIs (key performance indicators), or MQLs (marketing qualified leads), having the data already cleaned and refined opens up the door to do what smart businesses do with these functions: make data-driven decisions that help establish a competitive edge, or improvement on existing process.
However, just because data lakes are composed of unrefined pieces of a picture doesn’t mean they’re without massive value. Raw data becomes valuable when you need to ask questions that haven’t come up before. It allows for quicker modeling and is better for exploratory analysis. “How is that?” you might be wondering. To answer that, let’s take it back to childhood days.
Whether you were a childhood geek like us, or a passerby, chances are you played with Legos at some point in your life. Typically there were two ways to play with Legos; one, you would get a box set and carefully follow the instructions to achieve the perfect outcome pictured on the front of the toy box. Or two, you had a hodgepodge of Lego pieces which you could use your imagination to convert to anything. To tie this together with the concepts we’ve laid out today…that bucket of random pieces can be viewed as your data lake. You can build just about anything with it, but it’s not that sensible to start with. Your finished Lego model would be your data warehouse; easy to make sense of and look at, but restrictive in terms of building something new or different. Lastly, the data lakehouse could be thought of in terms of a combination of the two; completed Lego models, and the additional pieces you need to add to, reconstruct, or build next to the already completed pieces.
All of these aspects play a crucial role in business operations, and the insights into them. Here at Kodda, we help you obtain quicker insights from all the above, which equips you to make faster and better decisions for your business. Click here to see our software in action!